Paiheng Xu
徐 徘 衡
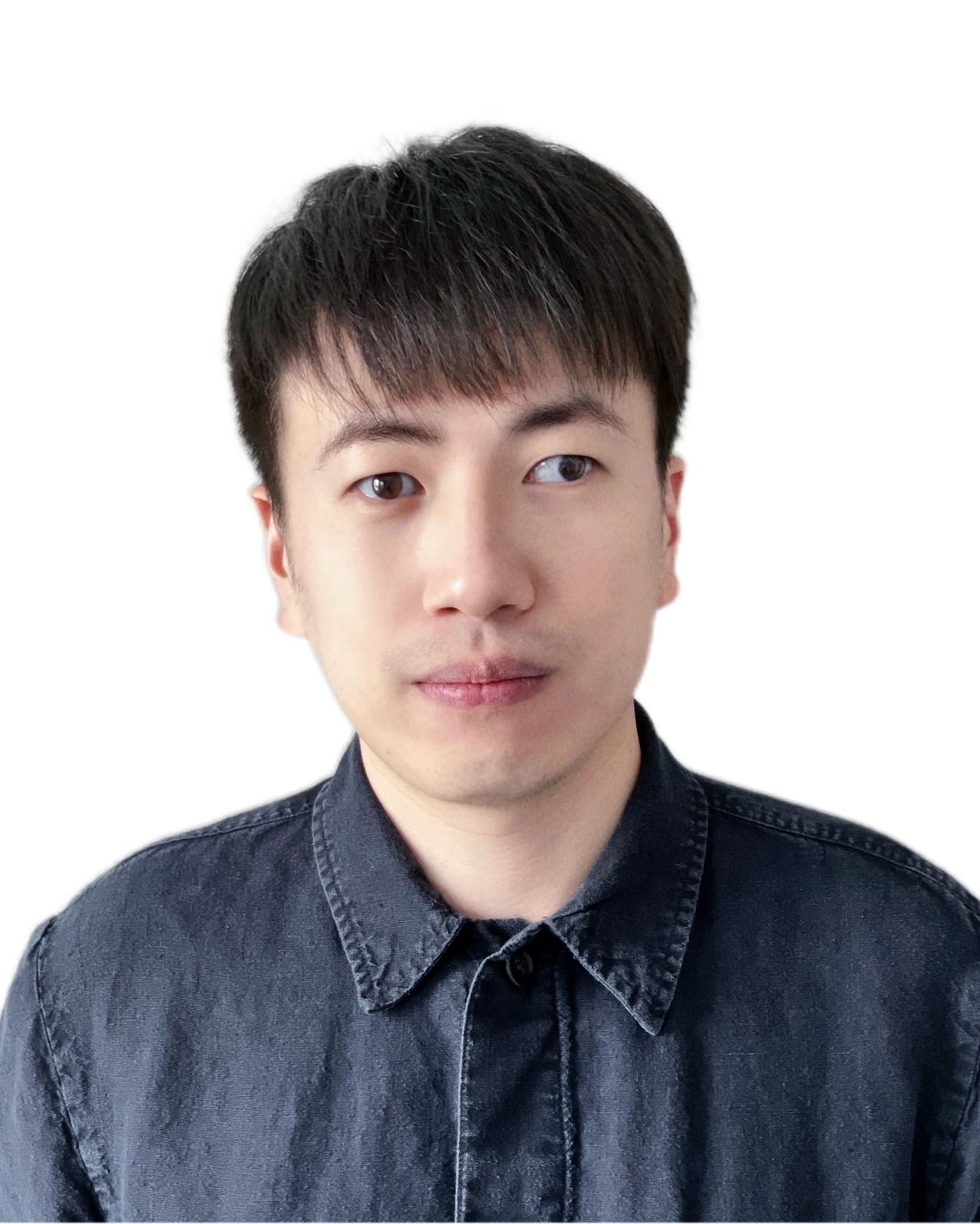
8125 Paint Branch Dr, Room 4104
College Park, Maryland 20742
I am a fourth-year CS Ph.D. student at the University of Maryland’s Computational Linguistics and Information Processing (CLIP) lab, advised by Wei Ai. My research interests are Natural Language Processing (NLP) and Computational Social Science, focusing on education and social media contexts. I am interested in understanding human conversations and developing applications using machine learning and NLP models within these domains, with considerations of causality and fairness. At UMD, I also closely work with Jing Liu, Louiqa Raschid, Vanessa Frias-Martinez, and Furong Huang
In the past, I have interned at Adobe Research. Previously, I graduated from Johns Hopkins University with M.S.E in Computer Science, where I worked with Mark Dredze at Center for Language and Speech Processing. I obtained my B.E in Computer Science from Southwest University, where I worked with Yong Deng on Complex Network, and Tao Zhou on Human Mobility.
News
Mar 2025 | Our survey on Large Language Models and Causal Inference is accepted to the Findings of NAACL 2025. |
---|---|
May 2024 | Our paper on concept level spurious correlations for text classification, with Yuhang Zhou, is accepted to ACL 2024. |
Mar 2024 | Our paper The Promises and Pitfalls of Using Language Models to Measure Instruction Quality in Education is accepted to NAACL 2024. See you in Mexico City! |
Jan 2024 | Our paper Twitter social mobility data reveal demographic variations in social distancing practices during the COVID-19 pandemic is available on Scientific Reports! |